Linear Regression
Linear regression is a method used in statistics to model relationships between a single, scalar variable and one or more other variables. In the linear regression method, a model is estimated for the unknown parameters from the existing data using linear functions. These models are referred to as “linear models.” Linear regression can also refer to a model in which some median of the conditional distribution of a variable, given a condition, is expressed as a linear function. Similar to other forms of regression, the linear regression application focuses on the conditional probability of an outcome’s given variables, instead of joint probability distributions as seen in multi-variable analysis.
What Are the Practical Applications of Linear Regression?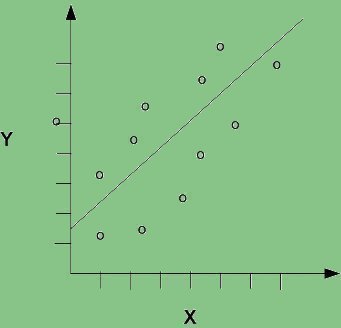
There have been a wide range of applications for linear regression over the past century. These applications can fit into two general categories:
- If there is a question that is forecasting or predictive in nature, linear regression techniques can be used to find a predictive model that can be fit to observed data to make a prediction of a second variable “Y” given the known value of the variable “X.”
- If a variable’s value and the associated values of related variables to this one is known, then linear regression may be used to find out how strong (if at all) the relationships between the variables and their subsets are in the problem.
A common misconception is that linear regression and the least squares approach are the same. They are actually used on dissimilar problems and are not the same. Linear regression analysis is used in behavioral and social sciences, biology, and in business to define or identify relationships between different variables.
What is Trend Estimation?
Trend estimation is when a trend line is used to show the movement over a time series of data and other variables. It can provide insights into the value of important data sets against time that may not be obvious if looked at separately, and are commonly determined by using linear regression in many business applications. It is typically used to argue that a dynamic event caused significant or observed change in a system at a given time. This technique does not use a control group, and is considered a simple analysis since it does not take into account other potential changes that could have impacted or changed the system at the time.
Comments - No Responses to “Linear Regression”
Sorry but comments are closed at this time.